Comparative Analysis of AI-Driven Machine Learning Models for Fault Detection and Maintenance Optimization in Photovoltaic Systems
DOI:
https://doi.org/10.51646/jsesd.v14i1.419Keywords:
Photovoltaic systems, machine learning, fault detection, maintenance optimization, Renewable energy.Abstract
With the increasing adoption of solar photovoltaic (PV) systems, ensuring their reliability and efficiency is crucial for sustainable energy production. However, traditional fault detection methods rely on expensive manual inspections or sensor-based monitoring, often slow and inefficient. This study aims to bridge this gap by leveraging machine learning techniques to enhance fault detection and maintenance optimization in PV systems. We evaluate five advanced machine learning models—Random Forest, XGBoost, Artificial Neural Networks (ANN), Convolutional Neural Networks (CNN), and Support Vector Machines (SVM)—using accurate operational data from a 250-kW PV power station. The dataset includes key operational parameters such as current, voltage, power output, temperature, and irradiance. Data preprocessing included outlier removal, feature selection via Pearson correlation, and normalization to improve model performance. The models were trained and tested using an 80-20 data split and evaluated based on classification accuracy, precision, recall, and F1-score. Our results show that XGBoost achieved the highest accuracy (88%), making it the best candidate for real-time predictive maintenance. Random Forest also performed well (87% accuracy), particularly in handling noisy data. ANN and CNN models effectively detected long-term degradation patterns, supporting preventive maintenance strategies. Based on these findings, we propose a dual maintenance strategy: XGBoost and Random Forest for real-time fault detection, while ANN and CNN monitor gradual system deterioration. This research provides a practical framework for integrating machine learning techniques into PV system management, offering a scalable solution to enhance reliability, reduce maintenance costs, and optimize energy efficiency.
Downloads
Metrics
References
M. Attia, N. Belghar, Z. Driss, and K. Soltani, "Automated Hydroponic System Measurement for Smart Greenhouses in Algeria," Solar Energy and Sustainable Development Journal, vol. 14, no. 1, pp. 111-130, 2025. DOI: https://doi.org/10.51646/jsesd.v14i1.306
D. Gielen, F. Boshell, D. Saygin, M. D. Bazilian, N. Wagner, and R. Gorini, "The role of renewable energy in the global energy transformation," Energy strategy reviews, vol. 24, pp. 38-50, 2019. DOI: https://doi.org/10.1016/j.esr.2019.01.006
M. Attia, M. Bechouat, M. Sedraoui, and Z. Aoulmi, "An Optimal Linear Quadratic Regulator in Closed Loop with Boost Converter for Current Photovoltaic Application," European Journal of Electrical Engineering/Revue Internationale de Génie Electrique, vol. 24, no. 2, 2022. DOI: https://doi.org/10.18280/ejee.240204
G. Di Lorenzo, R. Araneo, M. Mitolo, A. Niccolai, and F. Grimaccia, "Review of O&M practices in PV plants: Failures, solutions, remote control, and monitoring tools," IEEE Journal of Photovoltaics, vol. 10, no. 4, pp. 914-926, 2020. DOI: https://doi.org/10.1109/JPHOTOV.2020.2994531
M. Del-Coco, M. Leo, and P. Carcagnì, "Machine learning for smart irrigation in agriculture: How far along are we?," Information, vol. 15, no. 6, p. 306, 2024. DOI: https://doi.org/10.3390/info15060306
C. Saiprakash, S. R. Kumar Joga, A. Mohapatra, and B. Nayak, "Improved fault detection and classification in PV arrays using stockwell transform and data mining techniques," Results in Engineering, vol. 23, p. 102808, 2024/09/01/ 2024, doi: https://doi.org/10.1016/j.rineng.2024.102808. DOI: https://doi.org/10.1016/j.rineng.2024.102808
N. Gokmen, E. Karatepe, S. Silvestre, B. Celik, and P. Ortega, "An efficient fault diagnosis method for PV systems based on operating voltage-window," Energy Conversion and Management, vol. 73, pp. 350-360, 2013/09/01/ 2013, doi: https://doi.org/10.1016/j.enconman.2013.05.015. DOI: https://doi.org/10.1016/j.enconman.2013.05.015
A. Moussa and Z. Aoulmi, "Improving Electric Vehicle Maintenance by Advanced Prediction of Failure Modes Using Machine Learning Classifications," Eksploatacja i Niezawodność – Maintenance and Reliability, journal article 2025, doi: 10.17531/ein/201372. DOI: https://doi.org/10.17531/ein/201372
D. d. S. M. Freire, "Cellular Time Activation Networks, a novel approach applied to photovoltaic anomaly detection," Universidade do Porto (Portugal), 2023.
B. Taghezouit, F. Harrou, Y. Sun, and W. Merrouche, "Model-based fault detection in photovoltaic systems: A comprehensive review and avenues for enhancement," Results in Engineering, vol. 21, p. 101835, 2024/03/01/ 2024, doi: https://doi.org/10.1016/j.rineng.2024.101835. DOI: https://doi.org/10.1016/j.rineng.2024.101835
E. H. Sepúlveda-Oviedo, L. Travé-Massuyès, A. Subias, M. Pavlov, and C. Alonso, "Fault diagnosis of photovoltaic systems using artificial intelligence: A bibliometric approach," Heliyon, vol. 9, no. 11, p. e21491, 2023/11/01/ 2023, doi: https://doi.org/10.1016/j.heliyon.2023.e21491. DOI: https://doi.org/10.1016/j.heliyon.2023.e21491
T. Kavzoglu and A. Teke, "Predictive Performances of Ensemble Machine Learning Algorithms in Landslide Susceptibility Mapping Using Random Forest, Extreme Gradient Boosting (XGBoost) and Natural Gradient Boosting (NGBoost)," Arabian Journal for Science and Engineering, vol. 47, no. 6, pp. 7367-7385, 2022/06/01 2022, doi: 10.1007/s13369-022-06560-8. DOI: https://doi.org/10.1007/s13369-022-06560-8
S. F. Ahmed et al., "Deep learning modelling techniques: current progress, applications, advantages, and challenges," Artificial Intelligence Review, vol. 56, no. 11, pp. 13521-13617, 2023/11/01 2023, doi: 10.1007/s10462-023-10466-8. DOI: https://doi.org/10.1007/s10462-023-10466-8
K. Devi and M. Srivenkatesh, "Convolutional Neural Networks for Fault Detection in Grid-Connected Photovoltaic Panels," Ingenierie des Systemes d'Information, vol. 28, no. 6, 2023. DOI: https://doi.org/10.18280/isi.280619
N. Franić, I. Pivac, and F. Barbir, "A review of machine learning applications in hydrogen electrochemical devices," International Journal of Hydrogen Energy, vol. 102, pp. 523-544, 2025/02/10/ 2025, doi: https://doi.org/10.1016/j.ijhydene.2025.01.070. DOI: https://doi.org/10.1016/j.ijhydene.2025.01.070
A. A. Soomro et al., "Insights into modern machine learning approaches for bearing fault classification: A systematic literature review," Results in Engineering, p. 102700, 2024. DOI: https://doi.org/10.1016/j.rineng.2024.102700
S. Ghoneim, A. E. Rashed, and N. I. Elkalashy, "Fault detection algorithms for achieving service continuity in photovoltaic farms," Intelligent Automation & Soft Computing, vol. 30, no. 2, pp. 467-479, 2021. DOI: https://doi.org/10.32604/iasc.2021.016681
S. Kumar, V. Kumar, S. Sarangi, and O. P. Singh, "Gearbox fault diagnosis: A higher order moments approach," Measurement, vol. 210, p. 112489, 2023. DOI: https://doi.org/10.1016/j.measurement.2023.112489
O. A. Youssef, "An optimised fault classification technique based on Support-Vector-Machines," in 2009 IEEE/PES Power Systems Conference and Exposition, 2009: IEEE, pp. 1-8. DOI: https://doi.org/10.1109/PSCE.2009.4839949
A. N. Boruah, S. K. Biswas, and S. Bandyopadhyay, "Transparent rule generator random forest (TRG-RF): an interpretable random forest," Evolving Systems, vol. 14, no. 1, pp. 69-83, 2023. DOI: https://doi.org/10.1007/s12530-022-09434-4
A. H. Elsheikh, S. W. Sharshir, M. Abd Elaziz, A. E. Kabeel, W. Guilan, and Z. Haiou, "Modeling of solar energy systems using artificial neural network: A comprehensive review," Solar Energy, vol. 180, pp. 622-639, 2019. DOI: https://doi.org/10.1016/j.solener.2019.01.037
M. Jalal, I. U. Khalil, and A. u. Haq, "Deep learning approaches for visual faults diagnosis of photovoltaic systems: State-of-the-Art review," Results in Engineering, vol. 23, p. 102622, 2024/09/01/ 2024, doi: https://doi.org/10.1016/j.rineng.2024.102622. DOI: https://doi.org/10.1016/j.rineng.2024.102622
J. Verma, L. Sandys, A. Matthews, and S. Goel, "Readiness of artificial intelligence technology for managing energy demands from renewable sources," Engineering Applications of Artificial Intelligence, vol. 135, p. 108831, 2024/09/01/ 2024, doi: https://doi.org/10.1016/j.engappai.2024.108831. DOI: https://doi.org/10.1016/j.engappai.2024.108831
A. Mellit, O. Herrak, C. Rus Casas, and A. Massi Pavan, "A machine learning and internet of things-based online fault diagnosis method for photovoltaic arrays," Sustalani, A. Ghennioui, and M. El Aroussi, "A photovoltaic power prediction approach enhanced by feature engineering and stacked machine learning model," Energy Reports, vol. 8, pp. 1288-1300, 2022.
G. Naidu, T. Zuva, and E. M. Sibanda, "A review of evaluation metrics in machine learning algorithms," in Computer science on-line conference, 2023: Springer, pp. 15-25. DOI: https://doi.org/10.1007/978-3-031-35314-7_2
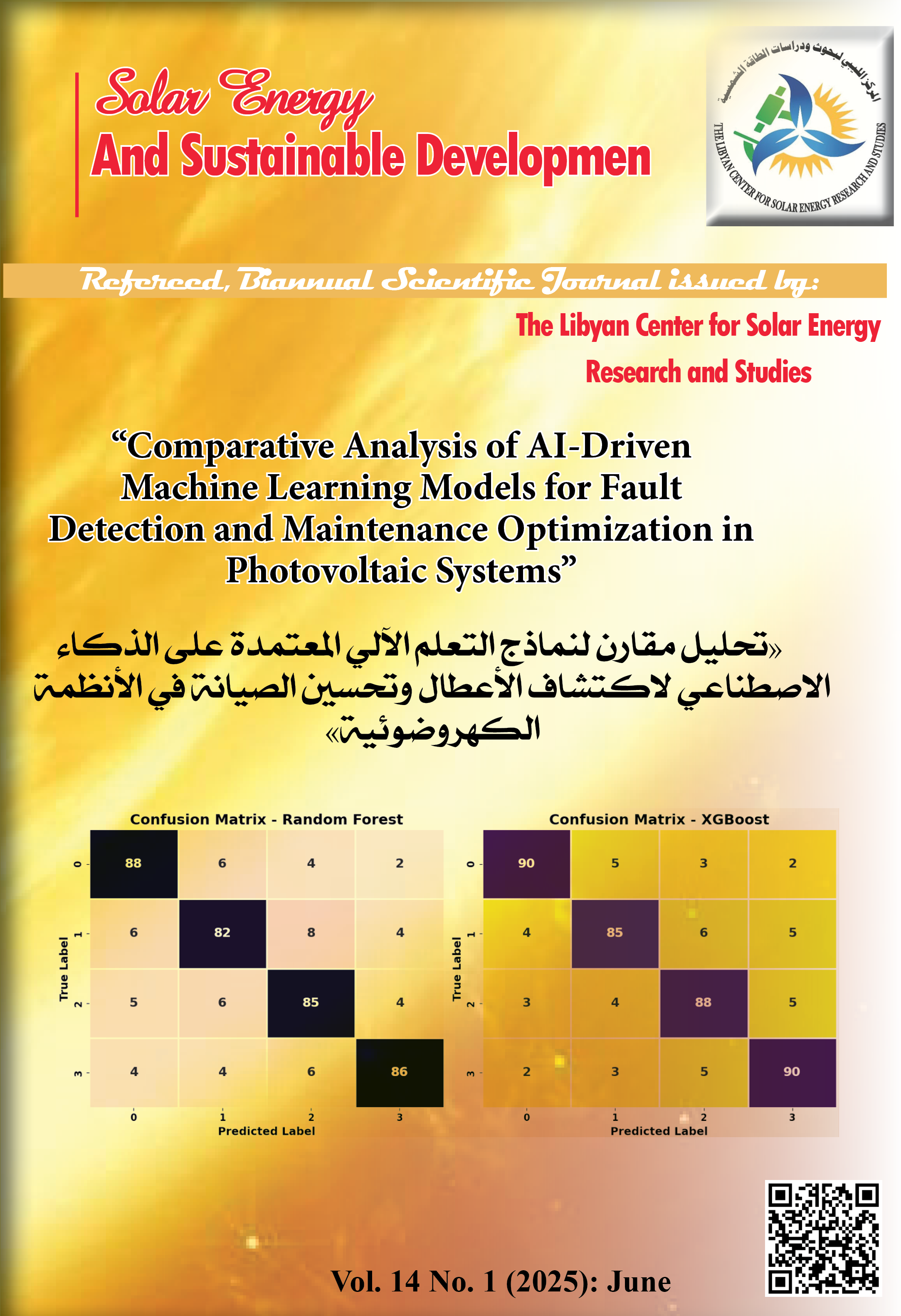
Downloads
Published
How to Cite
Issue
Section
License
Copyright (c) 2025 Solar Energy and Sustainable Development Journal

This work is licensed under a Creative Commons Attribution-NonCommercial 4.0 International License.